Volume 11 - Year 2024 - Pages 01-07
DOI: 10.11159/jbeb.2024.001
Cognitive Adaptation and Cortical Connectivity Alterations during Daily Tasks with Supernumerary Robotic Finger Assistance
Rateb Katmah1, Mohammad I. Awad1,2,5, Aamna Al Shehhi1, Feryal A. Alskafi1, Abdul Aziz Hulleck3, Herbert F. Jelinek4,5, and 01,5
1Khalifa University, Department of Biomedical Engineering & Biotechnology, AbuDhabi, United Arab Emirates.
2Khalifa University, Center of Autonomous Robotics Systems (KUCARS), Abu Dhabi, United Arab Emirates.
3Khalifa University, Department of Mechanical Engineering, AbuDhabi, United Arab Emirates.
4Khalifa University, Department of Medical Sciences, AbuDhabi, United Arab Emirates.
5Khalifa University, Healthcare Engineering and Innovation Center, AbuDhabi, United Arab Emirates.
100062079@ku.ac.ae; mohammad.awad@ku.ac.ae; aamna.alshehhi@ku.ac.ae; 100057997@ku.ac.ae; 100052964@ku.ac.ae; herbert.jelinek@ku.ac.ae; kinda.khalaf@ku.ac.ae.
Abstract - In light of the increasing incidence of upper-limb motor disorders, especially following strokes, there is a growing focus on developing assistive technologies to improve patient independence. This study investigated the effect of using a supernumerary robotic finger (SRF) on cortical functional connectivity during daily activities. SRFs have shown potential in overcoming grasping difficulties by employing adaptive strategies for manipulating various objects. To evaluate the neural responses to SRFs, neuroimaging methods such as fMRI and EEG were utilized. This research introduced a novel method by analyzing cortical functional connectivity through EEG sub-band analysis of the beta-band using Phase Locking Value (PLV) during simple daily tasks. The findings reveal task-specific changes in cognitive processing associated with SRF use. These results highlight the complex interaction between external assistive devices and shifts in cortical functional connectivity, offering important insights for developing more effective assistive technologies.
Keywords: Supernumerary robotic finger, Phase locking value, Functional connectivity, EEG.
© Copyright 2024 Authors This is an Open Access article published under the Creative Commons Attribution License terms. Unrestricted use, distribution, and reproduction in any medium are permitted, provided the original work is properly cited.
Date Received: 2024-03-02
Date Revised: 2024-09-01
Date Accepted: 2024-09-15
Date Published: 2024-09-25
1. Introduction
Upper limb movement and hand/finger directed movement are of paramount importance in their interaction with the environment, as they facilitate the manipulation of diversely sized and shaped objects, as part of the response to our immediate personal space consisting of cognitive and emotive adaptation. Recently, there has been a notable increase in post-stroke cases, attributed to the rise in aging population. More than 80% of individuals with hemiplegia following a stroke experience varying degrees of upper-limb sensorimotor dysfunction due to damage to the central nervous system [1]. These motor disorders, particularly those affecting the upper limb, significantly impact ability to perform activities of daily living (ADLs). Consequently, researchers are actively working on developing technologies to enhance patient self-sufficiency and promote independent living [2].
Numerous assistive robotic devices are currently addressing the challenge of limited hand grasping capabilities. One option involves utilizing a supernumerary robotic finger (SRF). The designs and applications of SRFs vary, with assistive SRFs designed for individuals with grasping difficulties often enabling an adaptive cylindrical grip. This is achieved through the incorporation of soft links/joints or other adaptive grasping mechanisms [3],[4], which are effective for grasping objects of varied sizes, except for very small items that demand a precise grip.
Neuroimaging methods such as functional magnetic resonance imaging (fMRI) and electroencephalography (EEG) enable the measurement of neural activity linked to the use of SRFs. These techniques unveil cognitive and emotive adaptations, showcasing activation, plasticity, and sensory integration patterns. Hussain et al. [5] noted higher neural activity when participants used an MRI-compatible extra robotic finger after training compared to before training. This increased activity was observed in various brain regions such as the cingulate cortex, superior and inferior parietal lobules, and middle frontal gyrus on both sides of the brain. Similarly, Mehring et al. [6] used fMRI to identify a unique cortical representation in polydactyl patients, revealing the brain's capacity to adapt to an extra limb, and enhancing manipulative skills. EEG, though limited to exploring cortical activity, can convey human intentions and adaptation for external device control. Liu et al. [7], using EEG and fMRI analyses, explored cerebral activity changes after a four-week SRF training, revealing significant alterations in cerebellar regions correlated with motor function improvements.
On the other hand, brain functional connectivity was investigated in a number of studies to quantify cognitive load. Zeng et al. [8] developed a system for visualizing cognitive activity with EEG, exhibiting brain connectivity across EEG channels and regions using Pearson correlation coefficient and coherence. Similarly, the effectiveness of integrating deep learning with EEG-based functional connectivity metrics in the classification of mental workload was emphasized by Gupta et al. [9]. Mazher et al. [10] found that coherence combined with graph theory-based network analysis outperformed traditional methods in assessing cognitive load using EEG data. Nevertheless, there is a gap in the literature concerning the analysis of cognitive load and mental states through EEG functional connectivity analysis when utilizing additional robotic limbs for everyday tasks.
Hence, this study presents a methodological approach for evaluating adaptation to the integration of an additional robotic finger from EEG patterns recorded from the EEG sensors. It employs beta sub-band EEG to examine brain functional connectivity, utilizing Phase Locking Value (PLV) for quantification. Through this technique, changes in cerebral connectivity induced by the performance of routine ADL tasks using the supernumerary robotic finger can be identified and analyzed. While PLV has been utilized previously for brain functional connectivity analysis, our study uniquely applies it in the context of assessing adaptation to robotic augmentation in ADL tasks. To refine the connectivity networks, we employed a data-driven thresholding approach based on Global Cost Efficiency (GCE), enhancing the precision of our findings regarding cerebral adaptation to the SRFs. As a result, the current findings will not only establish a fundamental basis for advancing knowledge regarding the effects of SRF usage on mental states and cognitive function of the user, but also facilitate advancements in the design and functionality of the SRF.
2. Methodology
2. 1. Participants
The study included ten Khalifa University students, five females and five males. The experimental procedure and study methodology were approved by Khalifa University's Institutional Review Board (Ref. H21-027). All of the subjects were healthy, right-handed, and had normal vision, hearing, and color perception, as well as no history of neurological dysfunction, substance use disorder or long-term prescription usage. They sat comfortably in a cool, airy room, where they received a detailed presentation about the experiment. Subjects completed informed consent forms prior to participation.
2. 2. Experiment Setup
The design of the SRF was based on a previous work [11], employing 3D printed rigid links (resembling finger phalanges) and soft links (mimicking joints). The rigid links are constructed from Polyactic Acid (PLA), while the soft links are made of Thermoplastic Polyurethane (TPU). The flexion-extension movement is achieved through a tendon-driven actuation system. A DC motor (Dynamixel MX-64) pulls the tendon for finger flexion, while antagonistic elastic rubber bands facilitate finger extension. The SRF was worn on the wrist of the dominant hand. A user-friendly push-button control system was implemented, allowing users to trigger flexion or extension with two buttons integrated into a wearable ring on the non-dominant hand.
EEG data were recorded using the Nexus-32 system (Mind Media, Herten, Germany). The cap was outfitted with 19 Silver/Silver Chloride wet electrodes arranged in accordance with the 10-20 international standard (Figure 1). Notably, the Nexus-32 does not use an electrode as a reference; instead, it employs a hardware common average reference. Data were collected at a sampling rate of 256 samples per second.
2. 3. Data Acquisition
The experimental design incorporated three distinct ADLs for the participants to complete: pouring water, sorting shapes, and a driving task on a driving simulator. Each subject underwent two recording sessions (phases). In the first phase, they performed the tasks using their dominant hand. In the second phase, the same tasks were repeated, but this time with an SRF affixed to their wrist. The order of these tasks was randomized among subjects.
For the "Pouring water" task, subjects were instructed to hold a 200-ml plastic bottle of water in their dominant hand (or SRF), pour the water into a glass, hold it, and drink from the glass. In the "Driving" task, participants drove for two minutes using a driving simulator. The steering wheel and paddles were linked to a Sony PlayStation 4 running a bus simulator game (created by Icebytes, Contendo Media, TML Studios, and Stillalive Studios). In the "Shapes sorting" task, subjects were asked to pick one object at a time with their dominant hand (or SRF) and insert it into the matching opening in the box. The experimental layout, comprising the three tasks and the two phases, is depicted in Figure 2.
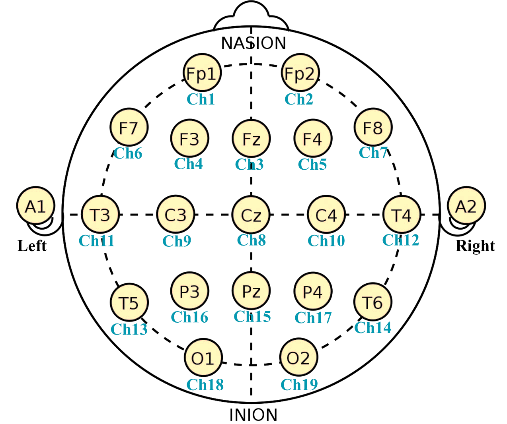
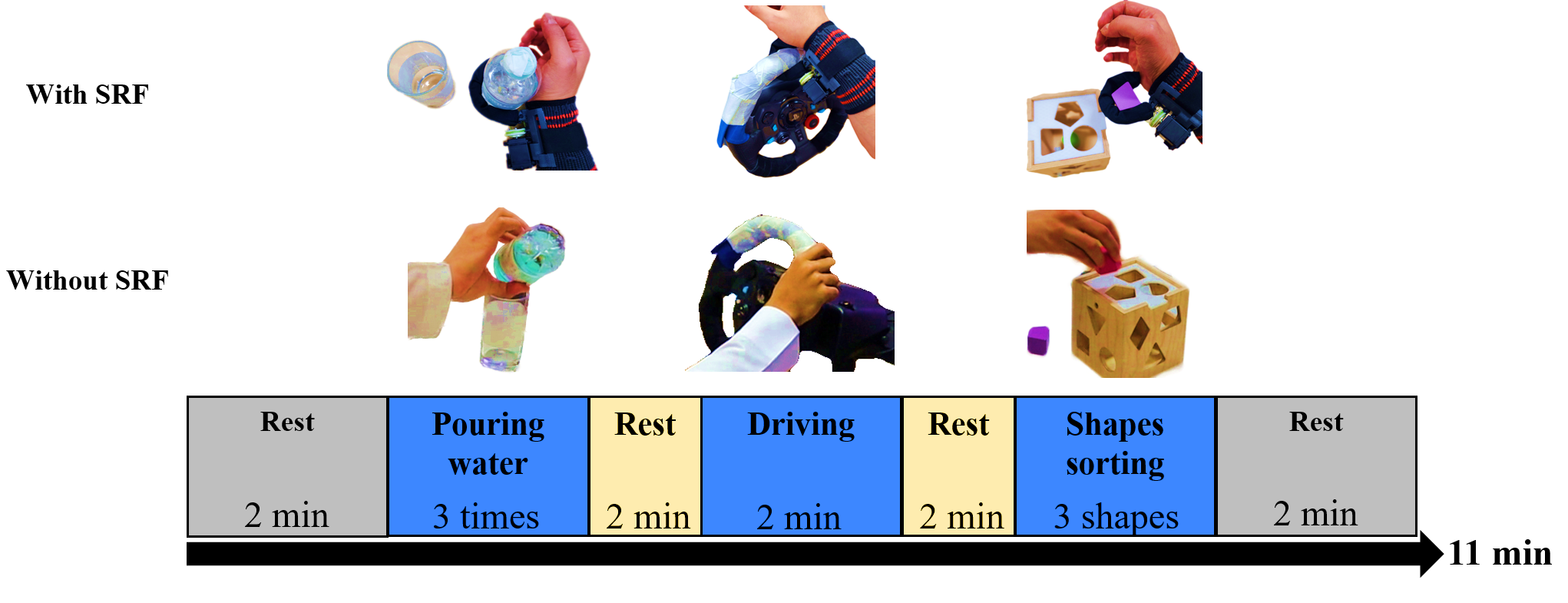
2. 4. EEG Preprocessing and Analysis
The raw EEG data were pre-processed using MATLAB R2022b and the EEGLAB v2021.0 toolbox to ensure data quality [12]. Band-pass filtering was applied with a passband of 0.5 Hz to 40 Hz. Subsequently, the signals were re-referenced to the common average reference. The Independent Component Analysis (ICA) method in EEGLAB was used to manually eliminate artifacts caused by eye blinks and movements. Following this, baseline subtraction was applied to the cleaned EEG data, and the signals were divided into target-related EEG epochs. Finally, the clean EEG data were divided into delta (0.5–4Hz), theta (4–8Hz), alpha (8–12Hz), beta (12–30Hz), and gamma (30–40Hz) frequency bands.
Functional connectivity was estimated by applying the Hilbert Transform to the bandpass-filtered epochs, utilizing the PLV [13]. As a result of its lack of reliance on presumptions regarding the data, the PLV is particularly suitable for the examination of non-stationary and non-linear signals [14]. Additionally, PLV permits the evaluation of phase coupling without the impact of zero-lag interference and is less sensitive to noise. In a time window, the PLV between channels u and v (PLVuv) is calculated as follows:
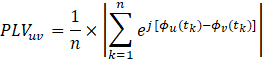
where, ϕu and ϕv represent the instantaneous phases of the EEG bands associated with channels u and v at time tk, respectively, and n denotes the time window length. To filter the connectivity networks, a data-driven thresholding strategy based on maximizing Global Cost Efficiency (GCE) was implemented. A 4D connectivity matrix was calculated for each individual and frequency band. This matrix included four key elements: phase, task, and the two electrodes connections involved. The matrix, therefore, shows the relationships between different pairs of electrodes. Analysis was limited to the EEG beta band due to its association with attention, cognitive processing, and mental effort as well as its high sensitivity to mental load state and stress [15].
Furthermore, since this research aimed to investigate how the brain adapts when utilizing SRF for daily living tasks, a statistical analysis was conducted between the two phases: phase 1 (without SRF) and phase 2 (using SRF). The Kolmogorov-Smirnov method was utilized to examine the normal distribution of the data. The evaluation of connection metrics between the phases was performed applying a two-sample t-test, employing the Bonferroni–Holm correction method to allow for multiple comparisons. Prior to the statistical analysis, all the statistical analyses were tested at the 95% significance level.
3. Results
In this study, we explored a novel approach to assess the brain's adaptation when integrating an additional robotic finger. We analyzed brain functional connectivity using PLV during daily living activities performed with the dominant hand (Phase 1) and repeated the analysis during the same activities with the added robotic finger (Phase 2). The robotic finger was activated by push-button with the contralateral hand. The investigation of Phase 1 provides insights into the impact of these activities on the EEG beta band functional connectivity, whilst the analysis of Phase 2 offers valuable indications about the influence of incorporating the robotic finger into the activities on the brain's adaptive processes.
Figure 3 displays the findings of the EEG beta band functional connectivity estimation using the PLV measure across both phases and all three tasks. PLV connectivity matrices were computed independently for each subject during phases 1 (without employing the SRF) and 2 (utilizing the SRF) before being averaged for all subjects. By employing GCE, weak connections in each task matrix were eliminated, retaining only actual connections. A decrease in PLV, indicates a weakening of the functional connection network, such as between the frontal and parietal-occipital lobes of the brain (shown as yellow and white nodes). Similarly, an increase in PLV indicates a corresponding expansion in the connectivity network, which paralleled the observed increase in connectivity among the frontal brain regions (red nodes).
Furthermore, the resultant phase 1 minus phase 2 connectivity network (Δ-GCE-PLV) underwent further filtration using a t-test at p<0.05. The matrix/experiment disparity, depicted in Figure 4 (a-c), is constrained within a range of -1 to +1. It depicts notable disparities in connectivity networks during the performance of the "pouring water" (Figure 4a), "driving" (Figure 4b), and "shapes sorting" (Figure 4c) tasks between the phases of "non-using" and "using" SRF. Channels exhibiting positive values (tending towards a red color) indicate a reduction in functional connectivity when the SRF was utilized. Conversely, negative PLV values (blue color) signify heightened functional connectivity within these brain regions when the SRF was employed for the specified task.
A notable rise in brain connectivity for EEG channels (F8, T4, and T6) when pouring water and using the SRF was observed, suggesting increased vigilance and reduced stress, as noted by Al-Shargie et al. [16]. In contrast, a decline in connectivity was noted between the memory function-related channel, T3 and T5 a cognitive processing channel Pz, and C3, which indicates sensorimotor integration. Combined the decreased activity in these channels may indicate heightened mental stress. Similar outcomes emerged in the driving task, with the T5 channel consistently showing reduced connectivity compared to other channels (O1, O2, T6, P3). However, for the "sorting shapes" task, the T4 channel displayed increased connectivity with cognitive processing / motor planning (F3), attention (Fp1), and judgment / coordination (Fp2) regions. This could be attributed to the nature of the task, involving actions such as grasping shapes correctly, deciding on matching holes, and orienting shapes for optimal fit.
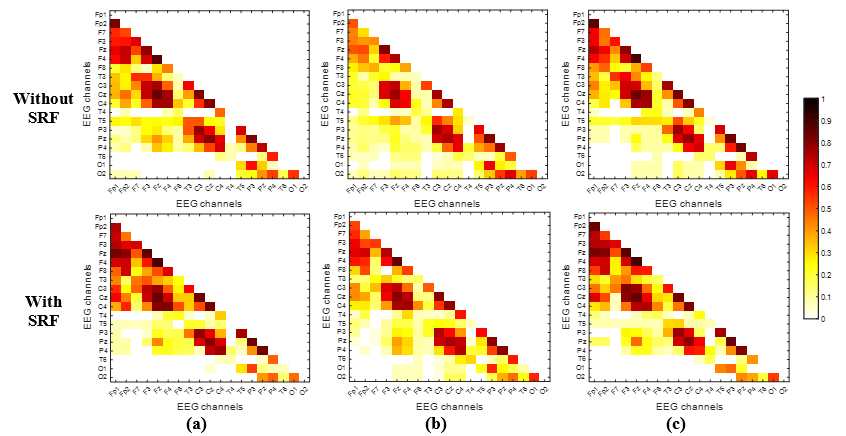
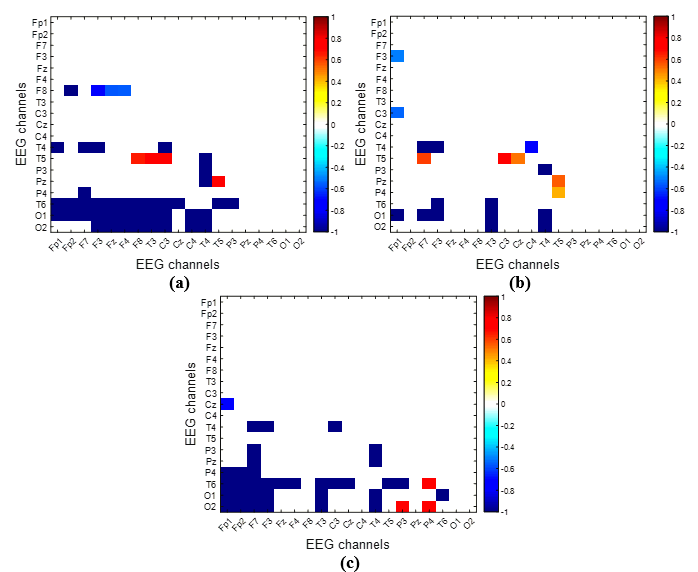
4. Discussion
Cortical phase synchronization and connectivity was significantly altered when a robotic finger was utilized to perform daily tasks, according to the EEG findings. Similarly, the decline in PLV weights observed in various brain regions indicates a state of reduced information transfer and processing both within and between cortical regions, suggesting that the ability to perform duties requiring constant vigilance will likely decline. This reduction is consistent with findings from [14] and [17], which similarly reported impaired connectivity in frontal and central regions during vigilance decrement and mental stress tasks. Study [14] found that vigilance decrement was associated with a frequency band-specific reduction in intra-regional connectivity, particularly within the frontal and central areas, aligning with our observation of reduced connectivity under the influence of the robotic finger. Moreover, [17] demonstrated that mental stress led to diminished information flow, particularly from the left to the right prefrontal cortex, mirroring the reduced connectivity observed in our study. However, unlike [17], which identified the role of binaural beats in enhancing connectivity to mitigate stress, our findings suggest that the robotic finger’s incorporation may have an opposite effect, further reducing connectivity as an adaptive mechanism against stress-induced cognitive impairment. Even so, study [18] showed that under certain mental stress situations, an increase in functional connectivity between cortical areas is occasionally observed. Hence greater connectivity could work as an adaptive mechanism against stress-related impairment of cognitive processes that subjects might have experienced while using SRF in the current research. This highlights the varied interplay between external tools, such as a robotic finger, and the brain’s functional connectivity, which could vary depending on the type of external stimulus or task at hand.
5. Conclusion
The findings of this study provide valuable insights into the cognitive adaptation when utilizing an additional robotic finger for daily tasks. The analysis of brain functional connectivity using PLV reveals task-specific alterations during SRF utilization. The observed changes in connectivity patterns suggest a delicate impact on brain dynamics, with implications for cognitive processing and mental stress. Specifically, changes in EEG characteristics may indicate changes invigilance and reduced stress during the water pouring task, while mental stress was highlighted in memory function-related channels during this task. Similarly, the driving task showed consistent reductions in connectivity, indicating potential implications for vigilance. The "sorting shapes" task displayed improved connectivity in cognitive processing, attention, and judgment regions, possibly reflecting the different nature of the task, which required more cognitive input and decision making. These findings underscore the complex interplay between external assistive devices and dynamic changes in the brain's functional connectivity, shedding light on the broader issue of brain adaptation in response to technological interventions. Further research is warranted to explore the long-term implications and potential applications of these findings in developing more effective assistive technologies for individuals with upper-limb motor disorders.
6. Acknowledgements
The authors would like to extend their gratitude to all participants who gave their dedication throughout the EEG recording process. The research was funded by Khalifa University of Science and Technology under Award No. CIRA-2021-106.
References
[1] F. Y. Wu and H. H. Asada, "Implicit and intuitive grasp posture control for wearable robotic fingers: a data-driven method using partial least squares," IEEE Transactions on Robotics, vol. 32, no. 1, pp. 176-186, 2016. View Article
[2] A. S. Ciullo, J. M. Veerbeek, E. Temperli, A. R. Luft, F. J. Tonis, C. J. W. Haarman, A. Ajoudani, M. G. Catalano, J. P. O. Held, and A. Bicchi, "A novel soft robotic supernumerary hand for severely affected stroke patients," IEEE transactions on neural systems and rehabilitation engineering, vol. 28, no. 5, pp. 1168-1177, 2020. View Article
[3] I. Hussain, L. Meli, C. Pacchierotti, and D. Prattichizzo, "A soft robotic supernumerary finger and a wearable cutaneous finger interface to compensate the missing grasping capabilities in chronic stroke patients," in 2017 IEEE World Haptics Conference (WHC), 2017: IEEE, pp. 183-188. View Article
[4] I. Hussain, M. Anwar, Z. Iqbal, R. Muthusamy, M. Malvezzi, L. Seneviratne, D. Gan, F. Renda, D. Prattichizzo, "Design and prototype of supernumerary robotic finger (SRF) inspired by fin ray® effect for patients suffering from sensorimotor hand impairment," in 2019 2nd IEEE International Conference on Soft Robotics (RoboSoft), 2019: IEEE, pp. 398-403. View Article
[5] I. Hussain, E. Santarnecchi, A. Leo, E. Ricciardi, S. Rossi, and D. Prattichizzo, "A magnetic compatible supernumerary robotic finger for functional magnetic resonance imaging (fMRI) acquisitions: Device description and preliminary results," in 2017 International Conference on Rehabilitation Robotics (ICORR), 2017: IEEE, pp. 1177-1182. View Article
[6] C. Mehring, M. Akselrod, L. Bashford, M. Mace, H. Choi, M. Blüher, A.-S. Buschhoff, T. Pistohl, R. Salomon, A. Cheah, O. Blanke, A. Serino & E. Burdet, "Augmented manipulation ability in humans with six-fingered hands," Nature Communications, vol. 10, no. 1, p. 2401, 2019. View Article
[7] Y. Liu, S. Huang, Z. Wang, F. Ji, and D. Ming, "Functional Reorganization After Four-Week Brain-Computer Interface-Controlled Supernumerary Robotic Finger Training: A Pilot Study of Longitudinal Resting-State fMRI," Frontiers in Neuroscience, vol. 15, p. 1870, 2022. View Article
[8] H. Zeng, Y. Jin, Q. Wu, D. Pan, F. Xu, Y. Zhao, H. Hu, W. Kong, "EEG-FCV: An EEG-Based Functional Connectivity Visualization Framework for Cognitive State Evaluation," Frontiers in Psychiatry, vol. 13, p. 928781, 2022. View Article
[9] A. Gupta, G. Siddhad, V. Pandey, P. P. Roy, and B.-G. Kim, "Subject-specific cognitive workload classification using EEG-based functional connectivity and deep learning," Sensors, vol. 21, no. 20, p. 6710, 2021. View Article
[10] M. Mazher, A. Qayyum, I. Ahmad, and M. O. Alassafi, "Beyond traditional approaches: A partial directed coherence with graph theory-based mental load assessment using EEG modality," Neural Computing and Applications, pp. 1-16, 2020. View Article
[11] I. Hussain, G. Salvietti, G. Spagnoletti, and D. Prattichizzo, "The soft-sixthfinger: a wearable emg controlled robotic extra-finger for grasp compensation in chronic stroke patients," IEEE Robotics and Automation Letters, vol. 1, no. 2, pp. 1000-1006, 2016. View Article
[12] A. Delorme and S. Makeig, "EEGLAB: an open source toolbox for analysis of single-trial EEG dynamics including independent component analysis," Journal of neuroscience methods, vol. 134, no. 1, pp. 9-21, 2004. View Article
[13] F. Al-Shargie, U. Tariq, M. Alex, H. Mir, and H. Al-Nashash, "Emotion recognition based on fusion of local cortical activations and dynamic functional networks connectivity: An EEG study," IEEE Access, vol. 7, pp. 143550-143562, 2019. View Article
[14] O. Hassanin, F. Al-Shargie, U. Tariq, and H. Al-Nashash, "Asymmetry of regional phase synchrony cortical networks under cognitive alertness and vigilance decrement states," IEEE transactions on neural systems and rehabilitation engineering, vol. 29, pp. 2378-2387, 2021. View Article
[15] F. Al-Shargie, S. Al-Ameri, A. Al-Hammadi, S. D. Vladimirovna, U. Tariq, and H. Al-Nashash, "Assessment of Mental Stress During 240-Days Isolation and Confined Environment using EEG Signals," in 2023 11th International IEEE/EMBS Conference on Neural Engineering (NER), 2023: IEEE, pp. 1-5. View Article
[16] F. Al-Shargie, R. Katmah, U. Tariq, F. Babiloni, F. Al-Mughairbi, and H. Al-Nashash, "Stress Assessment and Mitigation using fNIRS and Binaural Beat Stimulation," in 2021 IEEE International Conference on Systems, Man, and Cybernetics (SMC), 2021: IEEE, pp. 2678-2683. View Article
[17] R. Katmah, F. Al-Shargie, U. Tariq, F. Babiloni, F. Al-Mughairbi, and H. Al-Nashash, "Mental Stress Management Using fNIRS Directed Connectivity and Audio Stimulation," IEEE Transactions on Neural Systems and Rehabilitation Engineering, vol. 31, pp. 1086-1096, 2023. View Article
[18] F. Al-Shargie, R. Katmah, U. Tariq, F. Babiloni, F. Al-Mughairbi, and H. Al-Nashash, "Stress management using fNIRS and binaural beats stimulation," Biomedical Optics Express, vol. 13, no. 6, pp. 3552-3575, 2022. View Article